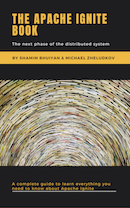
Apache Ignite offers several ways to improve a Spark job's performance: Ignite RDD, which represents an Ignite cache as a Spark RDD abstraction, and Ignite IGFS, an in-memory file system that can be transparently plugged into Spark deployments. Ignite RDD allows easily sharing states in-memory between different Spark jobs or applications. With Ignite in-memory shares RDDs, any Spark job can put some data into an Ignite cache that other Spark jobs can access later. Ignite RDD is implemented as a view over the Ignite distributed cache, which can be deployed either within the Spark job execution process or on a Spark worker.
Before we move on to more advanced topics, let's have a look at the history of Spark and what kinds of problems can be solved by Ignite RDDs.
Apache Spark was invented by AMPLab for fast computation. It was built on top of Hadoop MapReduce and extends the MapReduce model to efficiently use more types of operations, such as interactive queries and stream processing.
The main difference between Spark and Hadoop MapReduce is that during execution, Spark tries to keep data in memory, whereas Hadoop MapReduce shuffles data into and out of disk. Hadoop MapReduce takes significant time to write intermediate data to disk and read it back. The elimination of these redundant disk operations makes Spark magnitudes faster. Spark can store data (intermediately) into memory without any I/O, so you can keep operating on the same data very quickly.
In order to store data into memory, Spark provides special dataset named Spark RDD. Spark RDD stands for Spark Resilient Distributed Dataset. Spark RDD has fundamental components of the Apache Spark large-scale data processing framework. The following illustration shows iterative operations on Spark RDD.
Note that the above figure is obtained from the Spark documentation. Spark RDD is an immutable, fault-tolerant distributed collection of data elements. You can imagine Spark RDD as a Hadoop HDFS in memory. Spark RDD supports two types of operations:
- Transformations, which create a new dataset from existing one
- Actions, which returns a value by performing a computation on the RDD (as shown in the next figure)
Spark RDD is created through the use of Spark transformation functions. Spark transformation functions can create Spark RDDs from various sources, such as text files. In addition to creating Spark RDDs from the text files, Spark RDDs may be created from external storage such as RDBMS, HBase, Cassandra, or any other data source compatible with Hadoop input format.
Most of the time, Spark RDDs are transformed from one RDD to another new Spark RDD in order to prepare the dataset for future processing. Let's consider the following data transformations steps in Spark:
- Load a text file with airline names and arrival times for any airport in RDD1.
- Load a text file with airline names and flight delay information for any airport into RDD2.
- Join RDD1 and RDD2 by airline names to get RDD3.
- Map on RDD3 to get a nice report for each airline as RDD4.
- Save RDD4 to file.
- Map RDD2 to extract the information of flight delay for certain airlines to get RDD5.
- Aggregate the RDD5 to get a count of how many flights are delayed for each airline as RDD6.
- Save the RDD6 into HDFS.
Spark RDDs are utilized to perform computations on an RDD dataset through Spark actions such as
count
or reduce
. But there is a single problem with the Spark RDD: Spark RDD can't share between Spark Jobs or SparkContext because Spark RDD is bound to a Spark application. With native Spark distribution, the only way to share RDDs between different Spark jobs is to write the dataset into HDFS or somewhere in the file system and then pull the RDDs within the other jobs. However, the same functionality can be achieved by using Alluxio (formerly Tachyon) or Apache Ignite.
Apache Ignite's memory-centric architecture enables RDD sharing in a very efficient and effective way. Apache Ignite provides IgniteContext and IgniteRDD to share RDDs between Spark applications.
- IgniteContext: IgniteContext is the main entry point to the Spark-Ignite integration. To create an instance of an Ignite context, a user must provide an instance of SparkContext and a closure creating IgniteConfiguration (configuration factory). Ignite context will make sure that server or client Ignite nodes exist in all involved job instances. Alternatively, a path to an XML configuration file can be passed to IgniteContext constructor, which will be used to nodes being started.
- IgniteRDD: IgniteRDD is an implementation of Spark RDD abstraction representing a live view of Ignite cache. IgniteRDD is not immutable; all changes in the Ignite cache (regardless of whether they were caused by another RDD or by external changes in cache) will be visible to RDD users immediately. IgniteRDD utilizes the partitioned nature of Ignite caches and provides partitioning information to Spark executor. A number of partitions in IgniteRDD equals the number of partitions in the underlying Ignite cache. IgniteRDD also provides affinity information to Spark via
getPrefferredLocations
so that RDD computations use data locality.
In the next part of this series, we are going to install Apache Spark and do the following:
- Run the
wordcount
example to verify the Spark installation. - Configure Apache Ignite to share RDDs between Spark applications.
- Run Spark applications through Spark Shell to use Ignite RDD.
- Develop a Scala Spark application to put some Ignite RDD into the Ignite cluster and pull them from another Scala Spark application.
Comments